With the innovation of technology, testing methods also need to be advanced. Artificial intelligence (AI) is now entering into quality assurance (QA), transforming the way businesses perform software testing and automation. AI testing has emerged as a highly valuable asset for various organizations, providing advanced test automation and optimized QA procedures. Using AI in testing is highly effective when developers face many issues in manual testing. For example, a user-friendly AI system effortlessly performs and records user interactions with a web product. In just 10-15 minutes, this AI system could smoothly manage thousands of test cases regularly. AI can enhance efficiency and streamline processes. Now, let’s explore how AI tools significantly elevate their performance, leading to substantial improvements effectively in QA.
How can Organizations Start Using AI-driven Testing for QA Test Automation?
With AI organizations improve the quality of their software products and deliver better user experiences. To get started:
- Start with Identifying your Testing Needs and take a look at the current test suite and find out features and scenarios that benefit from automated testing.
- Research different platforms and Choose a Testing Platform that meets your requirements. Take into consideration features like user-friendliness, different test framework support and pricing.
- Establish testing processes that are consistent with AI-driven platforms for accurate outcomes. Defining test cases, creating automated tests, running tests and analyzing results should be included in this.
- Utilize the acquired insights for ongoing product Performance Monitoring, quick issue identification and initiate corrective actions when necessary. This guarantees a favorable user experience across various platforms.
- Making Improvements in tests as and when required will help ensure that tests remain current and accurately show present usage patterns, enabling you to maintain a competitive edge.
Features of AI with QA Test Tools
AI is effectively changing the way tasks are performed, enabling businesses and organizations to streamline operations, make informed decisions, and deliver more personalized services. In this discussion, we will explore the essential features that AI brings to the table and its impact on the landscape of technology and beyond.
Visual Testing and Monitoring
Artificial intelligence enhances the capabilities of testing platforms, with a specific focus on enhancing visual testing and support proficiency. The integration of AI into the Quality Assurance (QA) automation process serves as a valuable tool for aiding development teams in delivering products that are not only visually impactful but also characterized by quick and superior quality. Moreover, with technology in assistance, the results derived from regression testing efforts can easily be assessed.
Gathering of Requirements
Collecting requirements plays a vital role in every software development project, but it can be a challenging process without the support of AI-driven software testing. This task involves managing detailed responsibilities like monitoring deliverables, tracking approvals and generating reports. The utilization of technology can facilitate the understanding of complicated systems by predefined key performance indicators analysis.
Test Cases Reuse
It is challenging to use and reuse existing software development assets to create effective test cases. With the assistance of AI, there’s no need to be overly concerned as it significantly simplifies tasks, such as quick checking of applications, collecting essential data, measuring loading times and enhancing UI testing capabilities beyond expectations.
Identify Error
Users cannot perform essential functions of software due to error in the code. When there are more errors in the code, it becomes tough to find and fix them. But with AI in quality testing, quickly discover these issues, improve the testing process, and predict where things might go wrong. Users can be satisfied with this feature and save you money in the long run.
Exploratory Testing
Users can get the desired results using fewer resources and codes when use of AI in software testing is being implemented. AI systems operate with remarkable speed and can deal with simultaneous tasks such as learning, test design and execution. This technology efficiently identifies testers and detects system flaws with the help of intelligent assistants.
Code Coverage Optimization
Code coverage measurements detect which portions of your code have been verified by a test run and which parts remain untested. Maximum test coverage can be attained through the use of AI-driven testing. With the appropriate tools, 100% code coverage becomes a realistic objective.
Accuracy
When using manual testing methods, especially for repetitive tasks, it’s easy for quality engineers to make errors. For example, your team could potentially misinterpret a design notation or make errors in variable names. Here, integration of AI in QA testing enables more efficient handling of repetitive tasks with greater efficiency will ultimately raise the project’s level of accuracy.
Key Challenges of AI in Quality Engineering
The quality assurance landscape saw a major transformation with the implementation of automation tools and open-source frameworks. However, there are pros and cons of every innovation. Following are a few challenges to overcome when practicing AI into the technology landscape.
Data Quality : This is the major concern when AI is being used in QA. For training and performance of AI models, high-quality data are required. It might be challenging to collect and manage such data, especially for complex systems. The data collected by testers from user interactions, system performance, and defect occurrences. To ensure the AI model acquires accurate patterns, meticulous data cleaning and precise data labeling are necessary.
Reliability Concern: Here, some testers decline to use AI because they either don’t get a clear idea about how it works or are uncertain about its capabilities. That’s why it’s important to explain the pros and cons of using AI in QA, which can help build trust and confidence in its adoption.
Model Bias: It’s essential to recognize and rectify defects, irrespective of their type or severity. However, AI models have the potential to introduce bias through various ways, including the selection of training data, choices of algorithms and the methods used for evaluation.
Cost and Complexity: In the short run, using AI in quality engineering may be complicated and expensive as infrastructure and specialist knowledge are necessary. Employing AI models involves significant computational demands, often requiring specialized hardware and software resources. The complexity grows with the potential that testers training will be required to use AI tools and procedures effectively.
The Transformative Impact of AI on the Future of QA Engineering
The rise of AI has also made it possible to test software products in different environments and situations, enabling software testers to identify and resolve issues more efficiently. The future of QA engineering is bright, with AI playing a significant role in transforming the testing process, The good part of AI is the:
- Accuracy and Reliability: AI algorithms can analyze large amounts of data, like code changes, requirements, historical defects, to identify patterns and predict potential issues. This helpsQA engineers to focus on important areas to improve accuracy and reliability on software testing. It also helps to identify and address potential risks early in the development cycle (SDLC), reducing the chance of critical issues when software goes live.
- Test Automation: As mentioned earlier, AI-driven automation tools can improve the speed and effectiveness of software testing. They can create flexible priorities for test cases, allowing QA engineers to optimize their work processes and attain more comprehensive test coverage. With AI’s capabilities in QA activities, it can open the door to creating self-adjusting tests that can respond to software changes dynamically. This, in turn, cuts down on the time and resources needed for maintenance.
- Test Management: AI-based test management systems can automate various aspects of test case management, including test suite organization, test execution scheduling, and result analysis. With AI, QA engineers can optimize their testing efforts, enhance collaboration, and gain valuable insights from test data, leading to informed decision-making and improved overall software quality.
About VOLANSYS
VOLANSYS offers a wide range of services, with expertise in the field of Artificial Intelligence (AI). We focus on providing AI-based solutions, where businesses leverage smart technologies and develop advanced customized solutions that serve their specific needs. Our knowledge in AI, along with deep expertise in other domains, allows us to offer creative AI solutions that open up new opportunities and give them an edge in the AI-powered world.
Check out our success stories to learn more about our experiences with AI/ML. Take a look at our insightful AI/ML blogs written by our experts, based on the extensive knowledge and experience they have developed.
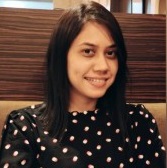
About the Author: Krupa Jagani
As a Marketing Executive at VOLANSYS, an ACL Digital Company, Krupa combines her passion for research with competence for crafting convincing content. Her valuable expertise lies in content creation and developing impactful marketing materials.